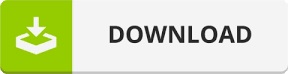
Suppose that the mean \( \mu \) is known and the variance \( \sigma^2 \) unknown.
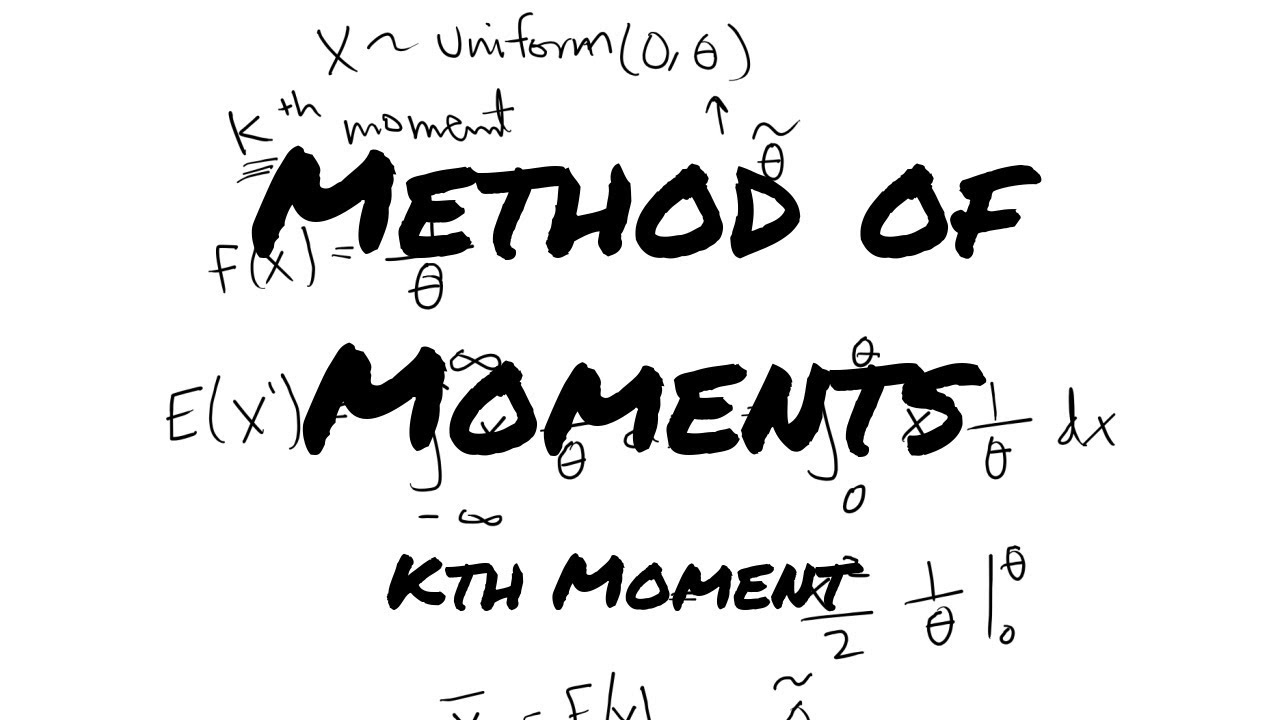
distribution has p unknown parameters, the method of moment estimators are. It may have no solutions, or the solutions may not be in the parameter space. MM may not be applicable if there are not su cient population moments. be known that the life of a lamp bulb has an exponential distribution with. In this case, take the lower order moments. If is the parameter of this distribution, then we have E(X 1) 1 On the other hand, the sample negative moment is: 1 10 + 1 13 + 1. For this distribution only the negative moments exist. Calculate the method of moments estimate for the probability of claim being higher than 12. It may have no solutions, or the solutions may not be in the parameter space. We want to t an inverse exponential model to this data. Next let's consider the usually unrealistic (but mathematically interesting) case where the mean is known, but not the variance. In this case, take the lower order moments. You cannot use the classical method of moments method to estimate parameters of a distribution without knowing the distribution and its parameters. Because of this result, \( T_n^2 \) is referred to as the biased sample variance to distinguish it from the ordinary (unbiased) sample variance \( S_n^2 \). Hence \( T_n^2 \) is negatively biased and on average underestimates \(\sigma^2\). The result follows from substituting \(\var(S_n^2)\) given above and \(\bias(T_n^2)\) in part (a).
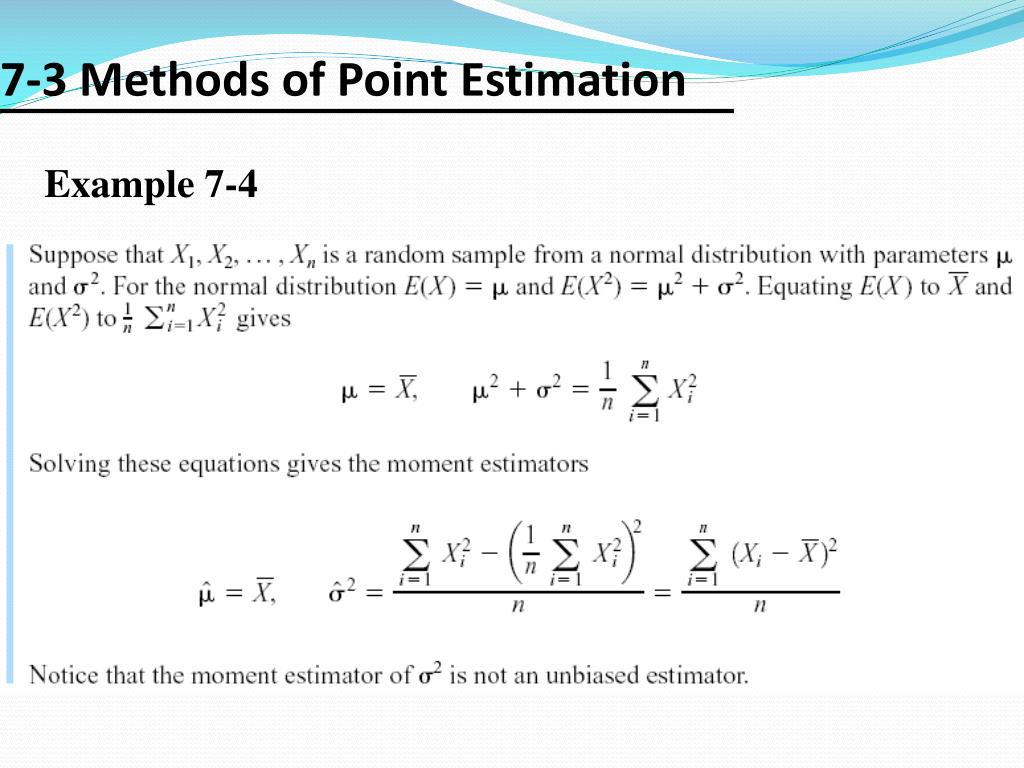
We also show the estimation using the PARETO_FITM function, as described in Real Statistics Support for Method of Moments.\right)^2 \var(S_n^2)\). Note, however, that since α ≤ 2, the variance is undefined (or is considered to be infinite). Since the estimated value of alpha is larger than 1, this approach is valid. We see from the right side of Figure 2 that α = 1.44977 and m = 1.01. The revised solution for Example 1 is shown in Figure 2 (only the first 8 data elements are shown). We, therefore, need to simplify the method of moments by settingĪnd estimating the value of alpha based on This means that this analysis is not valid. In the rst situation, there is no method of moments estimator. It might be the case that µ 1 µ 1 has no solutions, or more than one solution. Call the solution MOM,themethod of moments estimator of. Note too that if we calculate the mean and variance from these parameter values (cells D9 and D10), we get the sample mean and variances (cells D3 and D4).Īlthough the estimated value of alpha is larger than 2, there are some data elements (column A) that are less than the estimated value of m. The method of moments estimator of is the value of solving µ 1 µ 1.

We see from the right side of Figure 1 that α = 2.373892 and m = 1.884156.
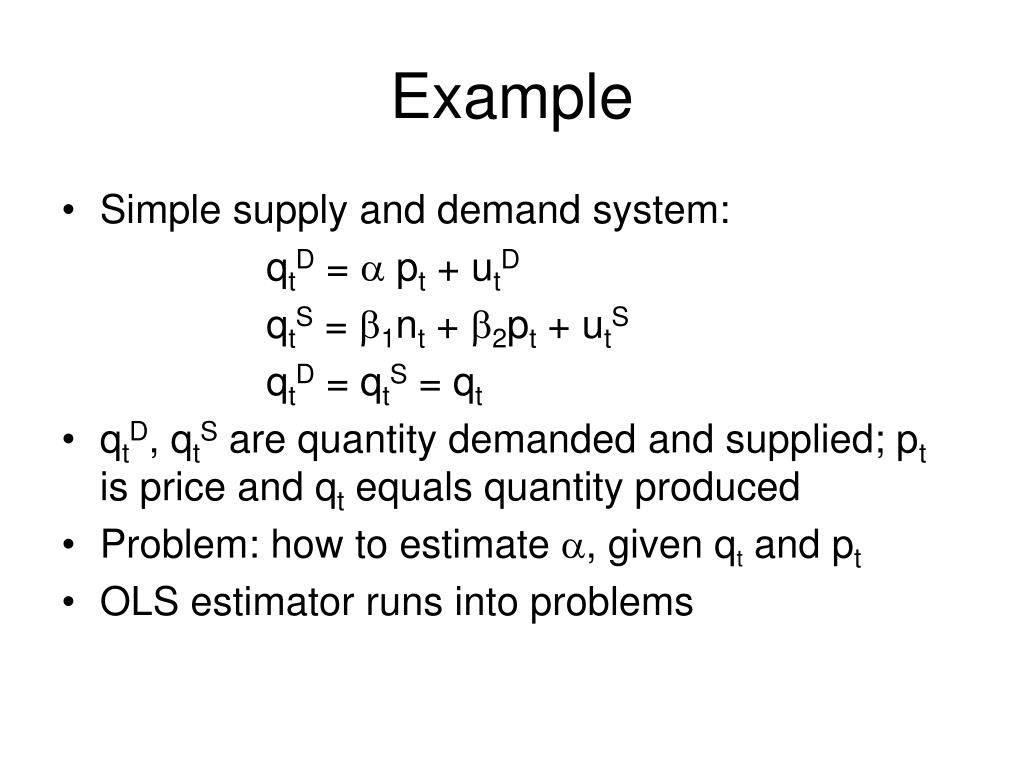
ExamplesĮxample 1: Determine the parameter values for fitting the data in range A4:A21 of Figure 1 to a Pareto distribution. We illustrate the method of moments approach on this webpage.Īs shown in Pareto Distribution, we can estimate the sample mean and variance for the beta distribution by the population mean and variance for α > 2 as follows:Įquating the two expressions for m and dividing by α–1, we getĬaution: The above analysis is only valid provided all the data elements in the sample are greater than or equal to m. Given a collection of data that may fit the Pareto distribution, we would like to estimate the parameters which best fit the data.
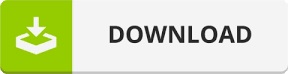